Durbin-Watson Test:
A popular test for detecting autocorrelation is the Durbin and Watson test statistic defined as
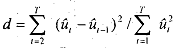
which is simply the ratio of the sum of squared differences in successive residuals to the overall error sum of squares (ESS).
If this was based on the true u, and T waq very largc then dcan be shown to tend in the limit as T gets large to 2(1-p). This means that if ρ->0, then d->2; if ρ->1 then d->0 and if ρ->1 then d-> 4. Therefore a test for II0, : ρ = 0, can be based on whether d is close to 2 or not. Unfortunately, the critical values of d depend upon the X, and these vary fiom one data set to another. To get around this, Durbin and Watson established upper (d0) and lower (dL) bounds for this critical value. It is obvious that if the observed d is less than the lower bound dL or larger I than (4 - dL), then we can safely reject Ho.
On the other hand if the observed d is between di, and (4 - d0) then we do no1 reject the hypothesis. If d lies in any of the two indeterminate areas, then one should compute the critical values depending on X. Most econometric software packages report the Durbin-Watson statistic automatically along with the p-value, that is the level of significance of this statistic (in general for the 5% level of testing if the p-value is less than 0.05 then you can reject the null hypothesis of no autocorrelation).
If we are interested in a one-tailed test, say Ho : p = 0 versus H1 : p > 0, that is positive autocorrelation only, then we would reject Ho if d < dL, and not reject Ho, if dL < dU. If on the other hand dL< d< dL then the test is inconclusive. Note that with a one tailed test only one side of the earlier test is operational. The conditions are still the same; the only difference is we ignore one set of conditions. With a two-tailed test we reject Ho based on both where d lies and where (4-4 lies. Here we are interested only in the value of d since we are testing only for positive autocorrelation versus the null of no autocarrelation. Earlier the alternate hypothesis was any type of autocorrelation. Similarly, for testing Ho : p = 0 versus H, : p < 0, we compute (44 and follow the same steps as before while testing for negative autocorrelation. Here our alterna~e hypothesis in only negative autocorrelation, versus the null of no autocorrelation.