We have mentioned earlier that in simultaneous models the explanatory variables and the error terms are not independent. So one of the important assumptions of OLS that there should be no covariance between the explanatory variable and the error term is violated. As a consequence the estimators are not consistent.
In order to show that the parameters are not conbistent, let us take a concrete example. Let us go back to the simple Keynesian model of income determination given in Example. Suppose we want to estimate the parameters of the consumption function given at eq. without taking into "account the income identity. As you know, the standard assumptions under OLS.
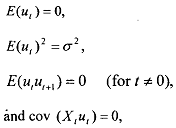
In the case of the consumption function we will first show that Yt and ut are correlated and then prove that OLS estimate is an inconsistent estimator of P,.
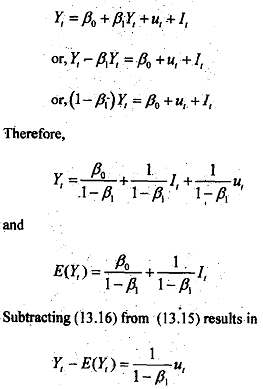
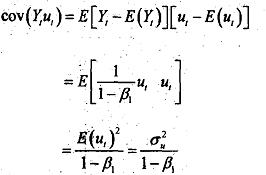
Since σ2 is positiveby defmition, the aovariance between Y and u given in is bound to be different fiom zero. Let us look back to eq i.e.,
Ci = β0+ β1Yt+ ut . Here, the explanatory variable Y, and the error term u, are correlated, which violates the assumption of the clissical linear regression model that the disturbaces are independent or at least uncorrelated with the explanatory variables. As noted previously, the OLS estimators in this situation are inconsistent.
To show that she OLS estimator .is' dnsistent because of correlation between Yt and ut , we ptoceed as follows:
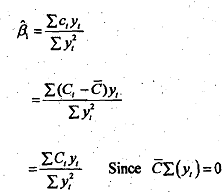
We know that the sum of the deviations ftom arithmetic mean is zero. Therefore, ∑Yt = 0.
Let us substitute in equation 4 for Ct . Thus we obtain
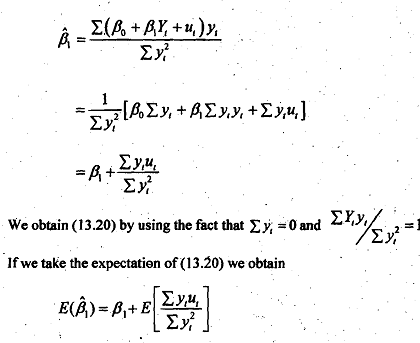
As expectation operator is a iinear operator, we cannot evaluate
. since
But intuitively it is clear that β1, will be unbiased only if
. Though we have shown in that the covariance between Y and u is nonzero that does not mean cov (ytut ) ≠ 0, since cov (yiui) is a population concept. It is not exactly equal to ∑ytut which is a sample measure, aIthough aq the sampk size increases indefinitely the latter will tend toward the fmmer, But if the sample size increases indefinitely, then we can resort to the concept of consist estimator and thus find out what happens to β1, as n, the smpIe size, increase indefinitely. In short when we cannot explicitly evaluate the expected value of an estimator, as in, we can turn our attention to its behavior in the sample.
Now an estimator is said to be consistent if its probablity limit, of plim for short, is equal to its tnrt (population) value. Therefore, to tow that P, of is inconsitennt: we must show that its plim is not equal to the true β1.
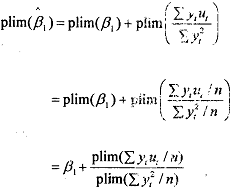
Where in the second step we have divided
by the total number of observations in the sample n so that the quantities in the parentheses are now the sample covariance between Y and u and the sample variance of Y , respective. In other words, states that the probability limit (plirn) of β1 is equal to true β1 plus the ratio of the plim of the sample covarance between Y and u to the plim of the sample variance of Y. Now as sample size n increases indefinitely, one would expect the sample covarince between Y and u to approximate the true population covariance E[Yt - E(yt)] [ut - E(ut)] which from is equal to

Similarly, as n tends to infinity, the sample variance of Y will approximate its 2 population variance, say σy2 . Therefore, equation may be written as
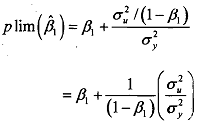
Given that O<β1 <.l and that σ2 and σy2 are both positive, it is obvious from equation that plim (β1) will always be greater than β1 that is, β1 will overestimate the true paramete, β1 in other words, β1 is a biased estimator, and the bias will not disappear no matter how large the sample size.