Important features of the dummy variables:
Before proceeding further it is essential to discuss some of the important features of the dummy variables which are as follows:
1) In the above models we have introduced only one dummy variable, D, to distinguish between two categories, male and female with D1 = 1 denoting maje and Di = 0 denoting female. Now what happens if instead of one dummy variable two dummy variables D1, and D2, are introduced in the model, one each for male and female? Model can now be written as .
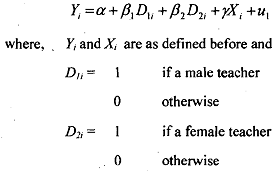
Due to perfect collinearity between D1and D2 (i.e., perfect linear relationship) model cannot be estimated. This can be more clearly explained with the help of the following data table.
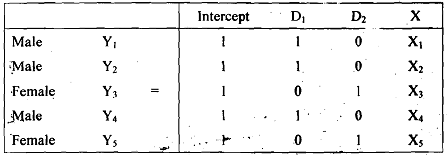
From the above table it is easy to verify that D1 and D2 are perfectly collinear as D1 = (1 - D2) or D2 = (1- D1).
There are, however, a number of ways of resolving this problem but the simplest one is by assigning the dummies as we had done in model and using orlly one .dummy variable if there are two categories of a qualitative variable.
Rule of Thumb: If a qualitative variable has m categories, introduce only (m -1) dummy variables Thus if a qualitative variable has 4 characteristics, introduce only 3 dummy variables. If this rule is not followed, we shall fall into what is known as the dummy variable trap, i.e., a situation of perfect multicollinearity.
2) The assignment of values 0 and 1 to two categories like rural and urban, or educated and uneducated etc., is arbitrary. For example in our model 10.5 instead of assigping 1 to male teacher and 0 to female teacher we could have assigned value 1 to female teacher and 0 to male teacher (and the coefficients would change accordingly). In such a case what is of importance is the interpretation of results. Thus in interpreting the results of the models that use dummy variables it is critical to know how the values 1 and 0 are assigned.
The category that is assigned a value 0 is often referred to as the base category or benchmark category and all the comparisons are made with reference to this category. In model female school teacher which is assigned value 0 is the base or benahmark category.
3) The coefficient attached to the dummy variable (for example, β in model) is referred to as the differential intercept coefficient because it tells by how much the value of the intercept term of the category that receives value 1 differs from that of the base category.