Definition of Multicollinearity:
First we take up the technical definition of multicdlinearity in the context of the Multiple Linear Regression Model (MLRM) and, then we will discuss a mare intuitive explanation of what it actually means for the researcher. Multicollinearity refers to the presence of aperfect, or exact, linear relationship among all or some of the explahatory variables in a regression. For instance if x1,...xn, (where X1 = 1 is the intercept term for all observations) are the variables being used in a regression, then an exact linear relationship between them is said to exist if the following condition is satisfied:

where A1,...,Ak are constants such that not all of them are zero simultaneously. Equivalently we can say that perfect multicollinearity implies that one of the variables can be exrpessed as a linear combination of the others, as shown below.
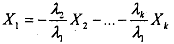
Exact linear relationship, how ever, is a pathological case and it almost never arises in the real world. This case also referred to as 'perfect multicollinearity' is therefore only usehl for clarifying the basic concept. In reality multicollinearity is commonly used to refer to a broader class of problems arising in MLRM, such that:

where v, is a stochastic (random) error term, that varies across observations. Note that for this case it is not possible to express any of the variables as a linear combination of the other explanatory variable due to the presence of the random (and therefore indeterminate term) v, for each observation. We will refer to this as the 'less than perfect multicollinearity' case. Although the implications of perfect multicollinearity are quite serious (see below), technically it is also the least bothersome as will be shown below. The more pervasive type of less than perfect multicollinearity that regularly arises in empirical studies is also technically the more troublesome since there is no easy way to avoid it.