Modelling, Planning and Scheduling of Manufacturing Processes
Process selection, Material selection, process sequencing, and machining parameter selection are the representative tasks covered in procedure planning. Scheduling and planning generally needs two steps as:
- The input-output process modeling and
- The selection of parameters for optimize the process along with describe constraints
On-demand flexible planning and scheduling can provide a very significant competitive benefit by decreasing waste, improving productivity and efficiency, meeting customer owing date, and reflecting the dynamic nature of more gradually competitive markets. Most scheduling and planning problems in manufacturing are NP-complete, along with precedence constraints in between tasks, timing requirements, setup costs, and completion deadlines. The shop management and scheduling is even difficult in flexible manufacturing systems along with on-demand productions. Classical heuristic techniques approach the problem by applying several priority rules based on several easily calculated job parameters, as like: due date, arrival times and setup times. Classical techniques obviously cannot acquire into account each variable interacting in manufacturing systems, and lack the time-dependent decision ability desired in production scheduling and planning, particularly in FMS and Computer Integrated Manufacturing or CIM environments that both need an ability to deal along with uncertainty and dynamic behavior. The capability of neural networks to know temporal patterns is necessary for efficient scheduling, planning and modeling of manufacturing processes.
In following figure depicts the applications of neural network to process scheduling, planning and modeling that include process selection, machine process optimization, process sequencing, and job scheduling. The neural network models utilized were as: multilayer feed-forward networks, Hopfield networks, MAXNET, ART networks and stochastic networks. The information acquisition abilities of neural network made them well-organized alternatives to traditional techniques for majority of scheduling and planning applications. The explanation's lacks for intrinsic casual associations exists in complex scheduling and planning applications and contain the demerits connected with the employ of neural network for applications. By combining the neural networks along with knowledge based systems as like: expert systems, these scheduling and planning problems can be efficiently solved along with neural networks.
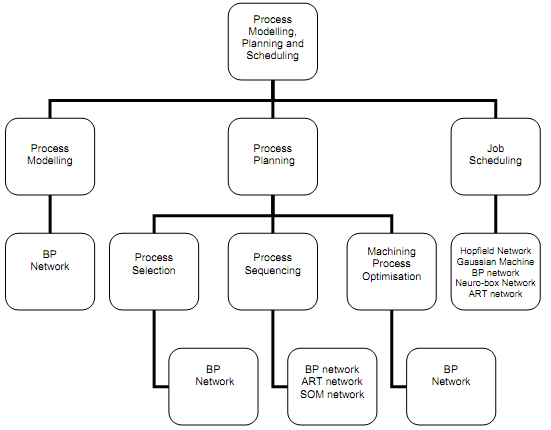
Figure: Hierarchy of Neural Network Applications for Process Modelling, Planning and Scheduling