Corrections for heteroscedasticity:
Once we have detected that there is heteroscedasticity in the error terms, we have to correct this problem. A simple method for correction of heteroscedasticity is the method of weighted least squares. We discuss this estimation technique in two conceptually separate cases based on our knowledge about the error variance. In the first case we assume that error variance is known in advance to us. In the second case we assume that the error variance increases or decreases with one of the explanatory variables.
i) When Error Variance is known
We assume thatsufficient prior knowledge is available for values of each of the error variances. The case of known variances occurs occasionally in econometric work but is important here in illustrating how to correct for heteroscedasticity. Recall that in the OLS method appropriate estimator is obtained by minimising the expression
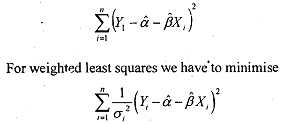
Thus each squared residual is weighted by
. Solving for the least-squares parameter estimates and using the variables in deviation form, we obtain
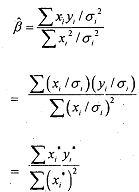
The desired estimation procedure is accomplished by weighting the original data and then performing ordinary least-squares estimation on the transformed model. Here the transformed model will be
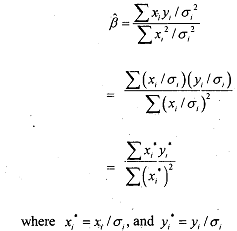
This procedure yields efficient estimators as the transformed model by construction satisfies all the assumptions of the classical linear regression model. A major difficulty with this estimation method, however, is that it involves prior information on the error variances which is not possible always. This dificulty, however, can be overcome by making certain assumptions about σ2. For example, we can estimate σ2 from the sample.