Consequences of heteroscedasticity:
Heteroscedasticity has two important implications for estimation. The first is that the least squares estimators, while still being linear and unbiased (in the case of finite but differing variances), are no longer efficient. They no longer provide minimum variance estimators among the class of linear unbiased estimators (that is, they are not BLUE). The second implicationls that the estimated variance of the least squares estimates is biased; so the usual tests of statistical significance, such as t and Ftests are no ionger valid. It is thus important to test for and remove heteroscedasticity from the data.
Let us discuss how the changing variance affects the desirable properties of regression coefficients. We will consider two aspects: unbiasedness and minimum variance of the OLS estimate of P. Let the model in deviation form be

Then the least squares estimator of B, is

By substituting the value of y, from (1) in the above equation (2) we obtsin
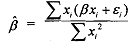
By rearranging terms in the above we have
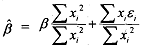
Under the standard assumption of E(E) = 0 , we find that

Notice that variances of the error terms play no role in the proof that least-squares estimators are unbiased and consistent. Thus Pis unbiased even in the presence of heteroscedasticity.
The problem lies with the variance of the estimated parameters p . The variance of b under the assumption of homoscedasticity, is

When there is heteroscedasticity the variance of p is quite different fiom (8.4). Let us derive the variance of b again.
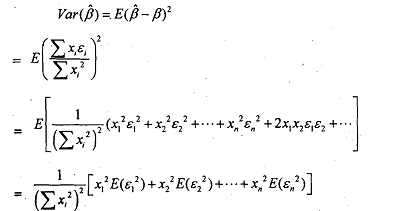
The variance of ci is not constant in the presence of heteroscedasticits Suppose that
and so on. In general terms we say that E(εi)2 = kσ2,By using the above in (8.5) we find that
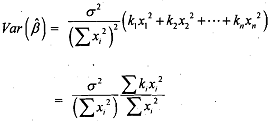
The difference between (8.4) and (8.6) is the factor
. If kt and x, are positively correlated and
then the classical least-squares estimation for the variance of ij will be overestimated. Thus the variance is different from that when the disturbance was homoscedastic. It means that the least squares estimates are not efficient (that is, not BLUE). It can
also be shown that the OLS estimates are not asymptotically efficient and therefore, the tests of significance and confidence limits do not apply.
Heteroscedastic disturbance, therefore, gives us false results whose significance is not liable to test. Hence, before testing for a hypothesis, the homoscedasticity of the disturbance term should be detected.