The Marginal Effects in the Logit Model:
As mentioned earlier the logit model is computationally more tractable than the probit model. The cumulative distribution function admits of a closed form (integral free). Consequently the expressions for the marginal effects are straightforward and easy to interpret. we deduce that the odds ratio for the logit model is
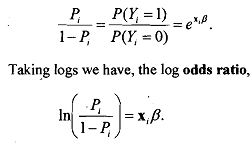
This is a linear function of the parameter vector 8. This makes the analogy with standard linear regression easier to comprehend. Differentiating gives us
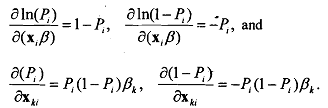
We could also have obtained these expressions directly by substitution for f(.) . Remember that Y, is a Bernoulli variable and V(Yi) = Pi(l- Pi). Therefore, the impact of a small change in the kth independent variable on the probability of the individual joining the'labor force is given by the variance of Y, times the coefficient β. The variance term on the right hand side of the expressions in captures the uncertainty arising from the lack of information on Y.