Interpretation of coefficient:
As in any nonlinear regression model the parameters of the logit and probit models, are not necessarily the marginal effects. This is in contrast to 'the interpretation of parameters in linear models we are accustomed to analyzing. The data available in these models is "limited", that is,hhe information available to us is less than in a. standard regression model. Therefore, we can no longer ask and answer the usual set - of quistions. All questions and answers now pertain not to the dependent.variable Y (or Y*) but to the probability of Y taking the value one or zero. In general,
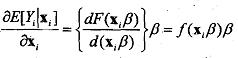
where A.) is the probability density function that corresponds to the (cumulative) distribution function, F(.).

While computing marginal effects, for making inferences, the expressions can be evaluated at the sample means of the data or evaluated at every observation and then averaged. The latter practice is favored by most researchers (provided the necessary regularity conditions are satisfied). Note also that the same scale factor applies to all the slopes in the model.
In addition,' one must be careful while computing the impact of dummy, independent variables on the probability of choice. The derivative is with respect to a small change, and it is not appropriate to apply for the effect of a change in a dummy variable. The correct marginal effect for a dummy independent variable, say d, would be given by:
Prob[Y = 1] d = 1, means of all other independent variables] - Prob [ Y = 1] d = 0, means of all otiiir independent variables]
The expressions obtained above are considerably simplified in the case of the logit model.