Hypothesis Testing and Goodness of Fit:
For testing hypotheses about the coefficients, all usual procedures are available. Tests on an individual coefficient will be based on the usual t tests, using the standard errors from the information matrix. The Wald test can be used to test a hypothesis about a subset of coefficients. Likelihood ratio and Lagrange multiplier statistics can also be computed. One of these procedures can also be used to test for heteroscedasticity or omitted variables. These tests are important as existing research indicates that the probit and logit models are not robust to the presence of heteroscedasticity or the omission of variables.
Several different measures have been suggested. The important thing to remember is that we are looking for a measure of how well the model approximates the obsewed data. The dependent variable being qualitative and binary, accuracy can be judged by looking at how well the calculated probabilities and the observed frequencies tally or by how well the model predicts observed responses.
Several measures analogous to the R2 measure for linear regression have also been proposed. McFadden has proposed the likelihood ratio index 
The measure is bounded between 0 and 1. If all the slope coefficients are zero then LRI equals zero. However, the LIU never attains the value 1.
Another popular tool available to us (one that should be used with caution) is to summarize the predictive ability of the model by forming a 2 x 2 table of the hits and misses of a simple prediction rule such as
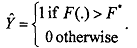
Usually F* is set equal to 0.5 or slightly higher. However, it is possible to.construct examples which make it clear that this tool is useful as a starting point but is not always accurate in evaluating models.