Instrumental variables method:
The problem of errors in the measurement of variables in regression models is quite important, yet econometricians do not have much to offer in the way of useful solutions. As a general rule, we tend to pass over the problem of measurement error, hoping that the errors are small enough which will not destroy the validity of the estimation procedure. One technique which is available and can solve the measurement error problem is the technique of instrumental variablys estimation.
We briefly outline the concept of instrumental variables because it is likely to be useful with measurement errors.
The method of instrumental variables involves the search for a new variable Z which is highly correlated with the independent variable X and at the same time uncorrelated with the error tern in the equations well, as the errors of measurement of both variables). In practice, we are conceined , ith the consistency of parameter estimates and therefore concentrate on the relationship between the variable Z and the remaining variables in the model when the sample size gets large. We define the
random variable Z to be an instrument . if the following conditions are met.
1) The correlations between Z and ε, u, and v, respectively, in the regression approach zero, as the sample size gets large.
2) The correlation bet&een Z and X is nonzero. as the sarnde size gets large.
We simply select one instrument (or combination of instruments) that has the highest correlation with the X variable.
Assuming for the moment that such a variable can be found, we can alter the lewt squares regression procedure to obtain estimated parameters that are consistent.
Unfortunately, there is no guarantee that the estimation process will yield unbiased parameter estimates. To simpli@the matter, let us consider the case of measurement krrors in the independelt variable such that yi = βxi + εi only x ismeasured with error (as x*= x + v). In order to solve the problem we take the regression equation yi = pzi + Where Z is the instrumental variable. The instrumental variables estimator of the regression slope in the above model is

The choice of this particular slope formula is made so that the resulting estimator will be consistent. To see this, we can derive the relationship between the instrumental-variables estimator and the true slope parameter as follows:
Clearly, the choice of Z as an instrument guarantees that will approach β as the sample size gets large [Cov (z, E*) approaches O] and will therefore be a consistent estimator of β. Remember that the variable x,' in (9.16) was not replaced by z, in the denominator of instrumental variables estimator, as the estimator does
not yield a consistent estimator of P. Let us show this.
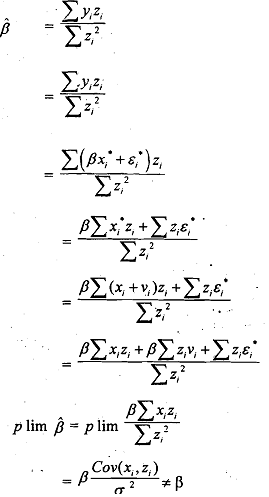
The above shows that
will not yield a consistent estimator of β.
The technique of instrumental variables appears to provide a simple solution to a difficult problem. We have defined an estimation technique, which yields consistent estimates if we can find an appropriate instrument.
A few concluding comments may be instructive.
i) First, the OLS. estimation technique is actually a special case of instrumental variables.: This follows because in the classical regression model X is uncorrelated with the error term and because Xis perfectly correlated with itself.
ii) Secorid, if we generalize the measurement error problem to errors in more than one independent variable, one instrument is needed to replace each of the designated independent variables.
iii) Finally, we repeat that instrumental-vdables estimation guarantees consistent estimation but does not guarantee unbiased estimation.