Nature of dummy variable:
In regression analysis the dependent variable is frequently influenced not only by variables that can be readily quantified on some well defined scale (e.g., income, output, prices, costs, weights, etc.), but also by other variables that are essentially qualitative in nature (e.g., marital status, gender, religion, cute, ee.). Shilariy studies in US have reported that female college teachers earn less than their male counterparts. Whatever may be the reason for this al.,parity, qwlitative variables like gender, institution of education, etc. do influence the dependent variable and should be included among the independent variables.
Since such qualitative variables usually indicate the presence or absence of some attribute or quality, such as rural or urban, male or female, married or unmarried etc. one can quantify such attributes by constructing artifical variables that take value 1 or 0,1 indicating the presence (or possession) of a particular attribute and 0 the absence of it or vice versa. For example, 1 ma) indicate that the person is a inale and 0 may indicate that the person is female; or 1 may indicate that a person is educated and 0 that he/she is not educated and so on. Such variables which assume values 0 .and 1 are called Dummy variables.
Like the quantitative variables the dummy variable can be used in regression analysis very - easily. In fact it may so happen that a regression model may contain only dummy explanatory variables. Regression models containing only dummy explanatory variables are called the analysis of variance (ANOVA) models. The following model is an example of ANOVA model
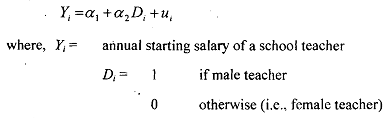
Model is like an ordinary two variable regression model. The only difference is the use of a qualitative or dummy variable D. instead of quantitative explanatory variable X. (From now on in the present unit we shall be using D to denote the dummy variable). Assuming all the other factors such as age, years of experience, etc. to be constant, the model may enable us to find out whether gender (i.e., being a male or female) makes any difference in a school teacher's salary. In other
words it would enable us to find out whether a male school teacher's salary is different from that of a female school teacher having the same qualifications and years of experience.
Assuming the d'igturbance term u, in the model satisfies the usual assumptions of the classical linear regression model (CLRM), we obtain from that mean salary of a female school teacher is:
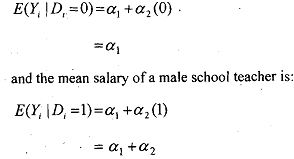
In the above model the intercept term α1 gives the mean annual salary of a female school teacher while the slope coefficient a2 tells US how much the mean salary of a male school teacher differs from that of his female counterpart with (α1 + α2) reflecting the mean annual salary of a male school teacher.
1 We can also test for the hypothesis: Is there a discrimination in accordance to the gender of an ihdividual while determining the salary of school teachers by running OLS on the regression equation and finding out on the basis of t-test whether the estimated α2 is statistically significant or not.