Auxiliary regressions:
Multicollinearity arises because one or more of the regressors are exact or approximate linear combinations of the other regressors. One way of finding out which X variable is related to other X variables is to regress each X, on the remaining X variables and compyte the corresponding R2, which we designate as R1 . Each one of these regressions is called an auxiliary regression; auxiliary to the main regression of Yon X's. There are subsequently two ways to test for multicollinearity:
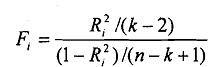
(a) First, we can follow lien's rule of thumb, which suggests that multicollinearity is a troublesome problem only if the R: obtained from an auxiliary regression is greater than the overall R2.
(b) Second, it can be shown that the variable
follows the F distribution with degrees of fkeedom k-2 and n-k+ 1. Here, n stands for sample size, k stands for the number of explanatory variables including the intercept term, and R1' is the explained variation from the regression of X on the remaining Xvariables. If the computed value of F exceeds the critical values at the chosen level of significance, then it is taken to mean that the particularx, is collinear with the otherxs. Conversely, if the computed F is lower than the critical value then we say that it is not collinear and we can retain it in the model.