Measurement Error in Y:
Let us assume that only the dependent variable contains error of measurement. Assume that the true regression model (written in deviation form) is

where E, represents errors associated with the specification of the model (the effects of omitted variables, etc.).
Assume that the variable y*, instead.of y, is obtained in the measurement process such that.

The measurement error u, is not associated with the regressor. Thus we have

The regression model is estimated with y' as the dependent variable, with no account being taken of the fact that y* is not an accurate measure ofy. Therefore, instead of estimating Eq. (9. I), we estimate
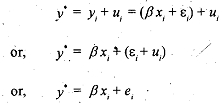
where ei is a composite error term, containing the population disturbance term ε, (which may be called the error term in the equation) and the measurement error term ui.
For simplicity let us assume that
(which is the assumption of classical linear regression), and Cov (xi, ui) = 0, i.e., the errors of measurement in yeare uncorrelated with xi, and Cov (εi, ui) = 0, th,e equation error and measurement error are uncorrelated. With these assumptions, it can be shown that P estimated from either (9.1) or (9.3) will be an unbiased estimator of the true P. Thus, the errors of measurement in dependent variable do not destroy the unbiased property of the OLS estimators. However, the variances and standard errors of P estimated from (9.1) and (9.3) will be different because, employing the usual formula, we obtain
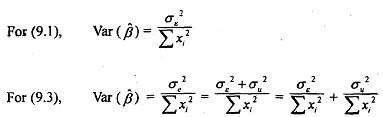
Obviously, the variance given at 19.5) is larger than the variance given at (9.4). Therefore, although the errors of measurement in the dependent variable still give unbiased estimates af the parameters and their variables, the estimated variances are now larger than the case where there are no such errors of measurement.