Presence of autocorrelation:
Note that the problem of autocorrelation primarily arises in the case of time series data and not for cross-sectional data. Panel data involves a different set of tools and are not covered in this course. It is easiest to make this point using the consumption function example. Say consumption and income data are collected across households (cross-sectional data) for August, 2006. It is extremely unlikely that the error terms will be correlated. That is secause correlation usually occurs when there is a common event that creates a deviation from the 'truey model. or 'hstance, one household holding a party may be a reason why their error term may be high (consumption expenditure much higher than predicted by income and other factors, which are the regressors or independent variables in the model estimated). However it is extremely unlikely that just because bouseliold i had a party, household j will also have a party during the same time period, when data was collected. Note that if all households are having a party during the survey period then this is a common factor for all data points and gets captured in the intercept term in the model (given income and other factors, consumption is predicted to be higher for all), and this does not affect the error terms.
For time series data, however, there is usually considerable inertia; most macroeconomic time series data follow a cyclical behaviour due to business cycles. For several periods income and consumption will be low; then income starts recovering. However, consumption shows an inertia and recovers after a lag as people become more confident that the rise in incomeis nJot temporary but permanent and change their consumption patterns slowly over time. In terms of the error term this will show up as several consecutive data points with low errors (deviations from the estimated model), then several periods when the errors are large (due to the inertia in economic behaviour), and so on.
In Fig.1, Fig.2 and Fig.3 we show different error terms, the true values, Note that the true values of error terms are not observable and are given here for illustrative purposes. In reality you will observe only the estimated residuals from the MRM. First, in Fig.1 there is no autocorrelation. In Fig.2, there is positive autocorrelation in the error structure. Finally, in Fig.3 there is negative autocorrelation. In the first figure you will find that the error terms are scattered around zero, which is expected, since it is a normal variable drawn independently with mean zero. Hence, there should be no correlation between consecutive error terms. So a plot should not reveal any pattern at all. In Fig.2 on the other hand there is substantial correlation between the error terins in consecutive periods; there are several periods when the error is high followed by several periods when it is low. So there is a cyclical pattern to be observed in the plot of residuals. This is true for most time series obtained from the real world, i.e., this is the most common pattern. Fig.3 shows negative autocorrelation. This shows period to period fluctuations, that is, if this period the error is highly positive the next period it will be highly negative and the period after that it will be highly positive again. In other words, consecutive terms are correlated but the sign changes from positive to negative and back and so on.
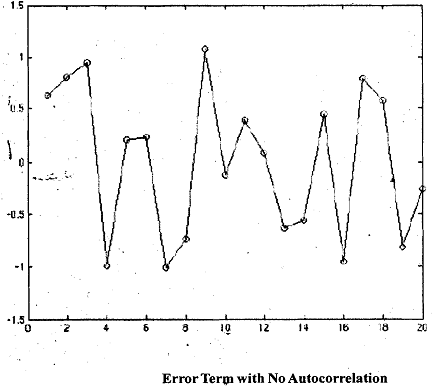
Fig.1
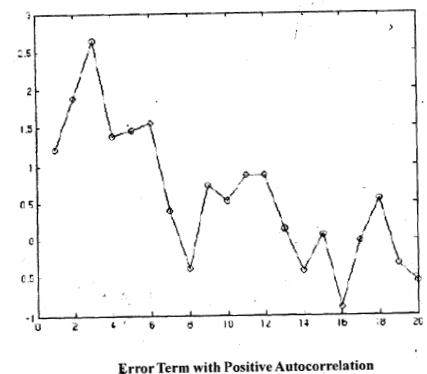
Fig.2
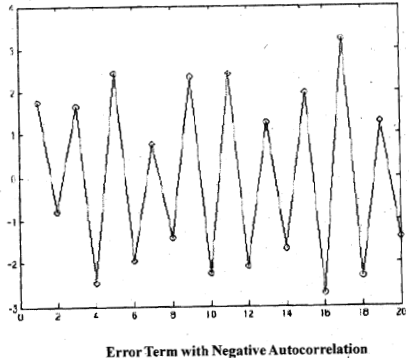
Fig.3