Autocorrelated Error:
To make this discussion more concrete, we now consider a specific example. In order to be more specific that we are dealing with time series data we use the subscript 't' instead of 'i'.

Let us assume that the error term is generated by the following mechanism
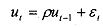
where p is also known as the coefficient of autocovariance and where E, is the stochastic error term such that it satisfies the standard OLS assumptions, namely,
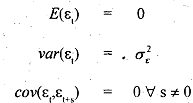
which says that &term has constant mean, fixed variance and is independent, not correlated with any othervalue of the time series Es. The technical term for the error generation scheme that we have assumed is aJirst order autoregressive scheme, usually denoted as AR(1) (more details on this are given in Unit on time series).Given this'scheme, it can be shown (derivations have been skipped for simplicity),
that
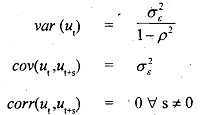
where corr(xy) denotes correlation between variables x and y. Note first that the variance of the error tern are fixed. Second, the error term is correlated not only with its immediate past value but also several period's past value as well. It is important to note that it always needs to be that |p|<l, that is, theabsolute value of p is less an one. If for example p=l then the variances and covariances above are not defined.' Under this assumption also note that the value of the covariance declines as we go further into the past. .
There are two reasons for using this process (first order autoregressive). First, it is the simplest form of a correlated error term structllre. Second, it is the most widely used in terms of applications. A considerable ammount of theoretical and empirical work has been done using such processes. The reasons for using the ,first order autoregressiGe process are both because it is the simplest form of a correlate&error term structure, as well as it is the most widely used in terms of applications. A considerable amount of theoretical and empirical work has been done using such processes.
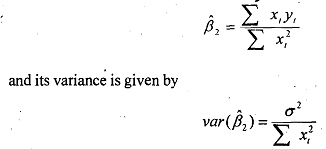
where yt is the deviation from the sample mean, that is yt = (yt - y') given that y' is the mean value of yt, and similarly for xi. Now it can be shown under this error scheme that the variance of the OLS estimator is

Note the difference between the variance of the OLS estimator under standard assumptions of no autocorrelation in the new variance of the estimator with autocorrelation (in the form of a first order autoregressive error term)'. The former is added by a factor which is the multiple of p as well as the sample autocorrelation between the values taken by the regressor X at various lags. Note that if p is zero then there is no autocorrelation which means that the two values will coincide as expected. We cannot say whether the former is smaller or bigger than the latter, but that they are different. Note in this context that for most economic time series data it is not unreasonable to assume that the regressors are positively correlated (consumption high in one period usually means it will be high in the next period as well), rarely do economic data follow a period to period fluctuations. Usually they follow a cycle (high values for a while 'during boom, followed by average and then low values during recession) wllich leads to positive correlation between successive values of the time series.