Over fitting Considerations - artificial intelligence
Left unexamined , back propagation in multi-layer networks may be very susceptible to over fitting itself to the training examples. The following graph plots the error on the training and test set as the number of weight updates increases. It is error prone of networks left to train unchecked.
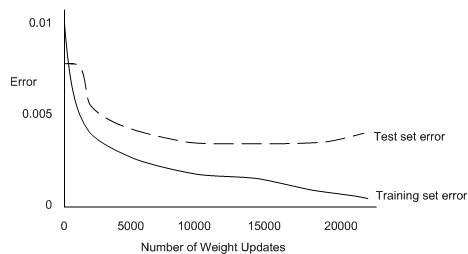
Alarmingly, even though the error on the training set continues to slowly decrease, the error on the test set essentially begins to increase towards the end. It is clearly over fitting, and it relates to the network starting to find and fine-tune to idiosyncrasies in the data, rather than to general properties. Given this phenomena, it would not be wise to use some sort of threshold for the error as the termination condition for back propagation.
In the cases where the number of training examples is high, one antidote to over fitting is to crack the training examples into a set to use to train the weight and a set to hold back as an internal validation set. This is a mini-test set, which may be used to keep the network in check: if the error on the validation set reaches minima and then start to increase, then it could be over fitting in beginning to occur.
Note that (time permitting) it is good giving the training algorithm the advantage of the doubt as much as possible. That is, in the validation set, the error may also go through local minima, and it is unwise to stop training as soon as the validation set error begin to increase, as a better minima can be achieved later on. Of course, if the minima are never bettered, then the network which is in final presented by the learning algorithm should be re-wound to be the 1 which produced the minimum on the validation set.
Another way around over fitting is to decrease each weight by a little weight decay factor during each epoch. Learned networks with large (negative or positive) weights tend to have over fitted the data, because larger weights are needed to accommodate outliers in the data. Thus, keeping the weights low with a weight decay factor can help to steer the network from over fitting.